Measuring to Manage
Chapter From
The Proceedings of the Workshop on"Reduction of Food Loss and Waste"Vatican City, 2020Scripta Varia 147ISBN 978-88-7761-115-4
Edited by:
Joachim von Braun, Marcelo Sánchez Sorondo & Roy SteinerIn partnership between the Pontifical Academy of Sciences and the Rockefeller FoundationBy: Steve Sonka
The Consortium for Innovation in Post-Harvest Loss and Food Waste ReductionProblem definition and relevance
In a world where more than 700 million people suffer from food insecurity and caloric deficiency, excessive post-harvest loss and food waste (PHL-FW) represents a missed opportunity to use food already present in the production system to reduce hunger, while increasing food quality/safety, nutrition, and market opportunities from small-holder farmers to consumers. Although data on actual PHL-FW are imprecise, the FAO (2011) estimated that approximately one-third of food produced for human consumption, 1.3 billion tons, is wasted or lost per year between farm and table. Subsequent estimates, from numerous sources in diverse settings, have affirmed that the magnitude of PHL-FW is significant (Flanagan et al., 2019.) Further, within the 2030 Agenda for Sustainable Development (SDGs,) Target 12.3 asserts the need to cut post-harvest loss by 50% by 2030 (United Nations, 2015.)
While the magnitude of loss today is concerning, it is even more distressing that excessive post-harvest loss and its associated negative impacts are NOT new topics of global interest. In 1975, then US Secretary of State, Henry Kissinger, made an eloquent plea detailing the need for global efforts to reduce post-harvest loss. Following his exhortation, the UN General Assembly adopted a resolution calling for at least a 50 percent reduction within ten years (Bourne, 1977). However, more than forty years later, we still struggle to find sustainable solutions to mitigate PHL of both cereals and perishables in developing countries.
In recent years, numerous technologies have been identified which can reduce PHL in developing countries (Flanagan et al., 2019). Documentation exists indicating that application of these technologies can reduce PHL and increase farmer profits (Fischler et al., 2011). Even though results of pilot efforts to reduce PHL have a history of positive outcomes, widespread adoption of the associated technologies is the exception rather than the norm (Easterly and Pfutze, 2008; Chandy et al., 2013).
Hanson et al. (2019) recommend that “countries and companies follow a simple ‘Target-Measure-Act’ strategy: adopt the Sustainable Development Goal target of halving food loss and waste as their own, measure their food loss and waste, and take action on the hotspots identified." As is often the case, the cost of actually measuring PHL is not rigorously examined. For many sectors in today’s economy, digital technologies have reduced dramatically the cost of measurement. However, in agricultural systems based upon smallholder farmers that is not the case and operational measurement can be costly.
In a recent conference statement, the Pontifical Academy of Sciences (2019) stresses that “while FLAW (food loss and waste) reduction has huge benefits, the costs of action cannot be ignored when aiming for effective and efficient solutions." This reality is particularly relevant with respect to measurement in smallholder farmer systems.
A corollary factor relates to the adequacy of information for decision-making purposes. For academic research, replicable accuracy is essential. While better accuracy is desirable, supply chain managers have to make decisions in a timely fashion. As noted by Bradshaw (2019), “actionable insights are the holy grail of any business’ data." Given the sparse nature of available data on post-harvest loss, emphasizing methods that can provide improved insights at very low cost is a worthy goal.
The purpose of this paper is to present an alternative approach to the concept of measurement of post-harvest loss, particularly in systems based upon smallholder farming. The paper’s following section will describe the approach. Then a numeric example is provided to illustrate application of the approach.
A more feasible approach to estimating loss
In some parts of food supply chains, measurement systems exist to monitor ongoing operations, for example, within modern food processing plants. In those settings, expanding the focus to include post-harvest losses often is economically feasible. However, on smallholder farms and in those parts of the food chain near the farm, measurement systems tend not to exist. Having to establish appropriate systems markedly increases the cost of physical measurement.
Assessing levels of post-harvest loss is not the only setting where continual, comprehensive measurement is not feasible. For example, estimates of the extent and nature of poverty are important factors affecting policy in developing countries. Continually and comprehensively quantifying poverty across populations would be a time- and resource-intensive undertaking. Attempting to do so would be quite costly.
Because policy makers need to understand the changing nature of poverty, methods have been developed which provide useful and cost-effective means to track poverty. In general terms a three-step process is employed:
Careful research is done to identify valid and quantifiable indicators of poverty. For example, an indicator could be the number meals the family consumes in a week.
Large-scale, representative, survey research is conducted. Such surveys, for example, could quantify the distribution of families in terms of the number of meals consumed weekly.
The sources o finformation in 1) and 2) are then combined to provide credible estimates of poverty. An important attribute of this approach is that estimates can be enhanced as additional survey information is obtained or as research provides improved poverty indicators.
The general approach just described is applicable as we attempt to understand post-harvest loss and to track the gains from interventions aimed to reduce loss. Again, a three-step approach would:
Conduct careful research, relating levels of loss to the alternative post-harvest practices employed. For example, hand-threshing rice is one practice. Use of mechanical threshers and combines are alternative practices. These post-harvest practices are analogous to the poverty indicators noted previously.
Large-scale surveys focused on quantifying the practices actually employed by farmers can be conducted to document their extent of use. Because these surveys would focus on what farmers actually do, recall-based responses are likely to be provided with minimal error.
As was the case for estimation of poverty levels, the sources of information in A) and B) can then be combined to provide credible estimates of post-harvest loss. To assess the effects of interventions aiming to reduce loss, surveys conducted over time would provide documentation of changes in the level of practices employed and therefore would allow decision makers to track changes in loss.
To this point, the discussion has focused on measurement of physical loss. However, it is important to note that physical losses are only one component of the economic effects of excessive post-harvest loss. Two key economic parameters associated with post-harvest loss are quality levels and the timing of sale of the farmer’s product.
The quality of the farmer’s output is often highly related to the practices employed on the farm and in transport of the product to market. For example, hand cut rice is often left for several days to dry in the field. The combined effect of heat in the day and dew overnight can reduce the desirability of the grain, therefore resulting in economic discounts at the market. Research efforts, such as noted in A) above, can assess the relationships between practices and quality to provide data needed to estimate economic losses because of quality deficiencies.
Further, if smallholder farmers don’t have the capability to adequately store or condition their output, they often suffer severe economic penalties by having to sell at harvest when market prices are typically at their lowest. This has been called PHL’s hidden tax (Sonka, 2014). For staple crops, this penalty is multiplied when the farm family must purchase the same product several months after harvest when prices are high.
Lack of access to appropriate post-harvest practices leads to substantial economic losses from both the effect of reduced quality and inappropriate market timing. In tracking the gains from reducing post-harvest loss, the effects of those parameters must be assessed, as well as the effect of physical losses on agricultural output. The framework described in this note can accommodate both of those factors.
An illustration
The following paragraphs illustrate application of the approach just described for a hypothetical, but realistic, post-harvest loss setting. The context is a rice supply chain from farm production to milling in Bihar state, India.
Figure 1 identifies five columns as relevant stages in the hypothetical supply chain. Three alternative pathways (the rows) are identified. At each node (intersection of supply stage and pathway), a numeric percentage is noted which represents an estimate of typical levels of post-harvest loss.
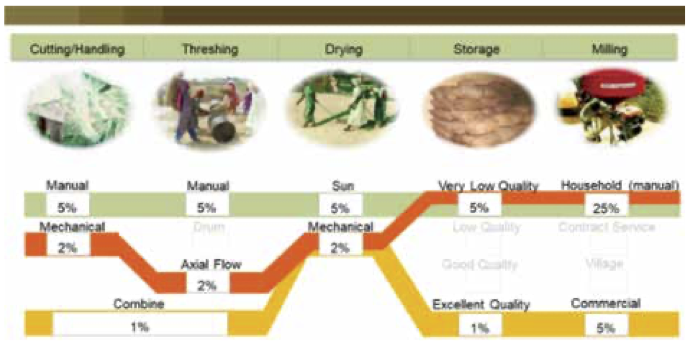
Three pathways are depicted in Figure 1:
The topmost pathway is representative of the traditional combination of practices, with hand-harvesting and threshing followed by sun-based drying and storage in jute bags.
The middle pathway differs from the traditional pathway by employing mechanical devices for harvest, threshing and drying.
The bottommost pathway incorporates combine harvesting,mechanical drying and high-quality storage and milling.
As noted previously, quality deterioration after harvest is an important economic factor. For purposes of this illustration, it is assumed that systems (pathways), which do little to protect quality, can expect 30% reductions in economic value at the end of the supply chain. Conversely, pathways which strive to maintain quality levels can expect only a 10% reduction in economic value.
The primary benefit of the proposed approach is that the framework is amenable to providing insights for the types of questions smallholder farmers, supply chain managers and policy decision-makers need to address. For example, a policy-maker or a supply chain manager might be interested in having a sense of the economic gains that could be achieved if the dominant system moved from traditional practices to the more modern practices of the bottommost pathway in Figure 1.
To further illustrate that application, let’s consider:
An agricultural district with 10,000 hectares of rice production,
Rice yields of 2,500 kg/ha in a good weather year,
An expected market price of 25,000 rupees per metric ton for high quality rice.
Using the parameters noted previously, it’s possible to compare the two
pathways. Economic estimates resulting from one such comparison include the following:
Prior to harvest, the potential economic value for both pathways is 625 million rupees.
The calculated economic value of the quantity losses is:
243.2 million rupees for the traditional pathway versus
188.6 million rupees for the improved pathway.
Further, the calculated economic value losses because of reduced quality are:
114.5 million rupees for the traditional pathway versus
43.6 million rupees for the improved pathway.
The resulting gross marketable value estimates are:
267.3 million rupees for the traditional pathway versus
125.5 million rupees for the improved pathway.
The numbers and estimates shown here are not meant to indicate the relevant value of the various practices mentioned. Rather they are solely meant to illustrate the type of framework and tools that decision-makers could employ to drive actions that can lead to reduced post-harvest loss.
In this illustration, the values linking loss levels to practices were based upon expert opinions. In reality, investigation of relevant research findings and more rigorous assessment of multiple sources likely would be conducted. While decisions generally need to be made at a point in time, decision-making in supply chains typically is a dynamic process. Experience over time can identify key decision points where additional research is critically needed to improve decision-making. Results from that research, combined with operational experience, can lead to better parameter estimates as well as improved decisions.
Concluding remarks
The Target-Measure-Act approach is an attractive means to articulate steps necessary to drive the change needed to reduce post-harvest loss, whether the actors are public or private decision-makers.This paper focus- es on the measurement step, noting that acquisition of accurate data within systems based upon smallholder farming is likely to be costly; cost levels which have the potential to exceed expected benefits.
An alternative approach is described and illustrated in this paper.The focus of the approach is production of insights that can enable better decision making. Further, explicit identification of key practices and their relationship to loss can drive learning through external research and operations.
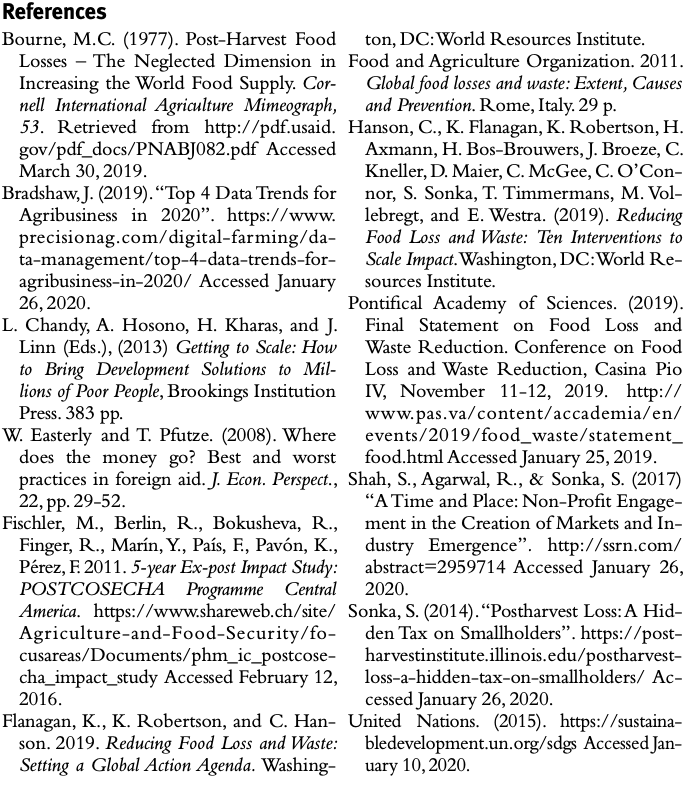